mirror of
https://github.com/paperless-ngx/paperless-ngx.git
synced 2025-04-02 13:45:10 -05:00
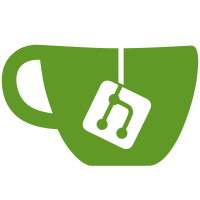
* Added devcontainer * Add feature storage pathes * Exclude tests and add versioning * Check escaping * Check escaping * Check quoting * Echo * Escape * Escape : * Double escape \ * Escaping * Remove if * Escape colon * Missing \ * Esacpe : * Escape all * test * Remove sed * Fix exclude * Remove SED command * Add LD_LIBRARY_PATH * Adjusted to v1.7 * Updated test-cases * Remove devcontainer * Removed internal build-file * Run pre-commit * Corrected flak8 error * Adjusted to v1.7 * Updated test-cases * Corrected flak8 error * Adjusted to new plural translations * Small adjustments due to code-review backend * Adjusted line-break * Removed PAPERLESS prefix from settings variables * Corrected style change due to search+replace * First documentation draft * Revert changes to Pipfile * Add sphinx-autobuild with keep-outdated * Revert merge error that results in wrong storage path is evaluated * Adjust styles of generated files ... * Adds additional testing to cover dynamic storage path functionality * Remove unnecessary condition * Add hint to edit storage path dialog * Correct spelling of pathes to paths * Minor documentation tweaks * Minor typo * improving wrapping of filter editor buttons with new storage path button * Update .gitignore * Fix select border radius in non input-groups * Better storage path edit hint * Add note to edit storage path dialog re document_renamer * Add note to bulk edit storage path re document_renamer * Rename FILTER_STORAGE_DIRECTORY to PATH * Fix broken filter rule parsing * Show default storage if unspecified * Remove note re storage path on bulk edit * Add basic validation of filename variables Co-authored-by: Markus Kling <markus@markus-kling.net> Co-authored-by: Trenton Holmes <holmes.trenton@gmail.com> Co-authored-by: Michael Shamoon <4887959+shamoon@users.noreply.github.com> Co-authored-by: Quinn Casey <quinn@quinncasey.com>
330 lines
11 KiB
Python
330 lines
11 KiB
Python
import hashlib
|
|
import logging
|
|
import os
|
|
import pickle
|
|
import re
|
|
import shutil
|
|
|
|
from django.conf import settings
|
|
from documents.models import Document
|
|
from documents.models import MatchingModel
|
|
|
|
|
|
class IncompatibleClassifierVersionError(Exception):
|
|
pass
|
|
|
|
|
|
class ClassifierModelCorruptError(Exception):
|
|
pass
|
|
|
|
|
|
logger = logging.getLogger("paperless.classifier")
|
|
|
|
|
|
def preprocess_content(content):
|
|
content = content.lower().strip()
|
|
content = re.sub(r"\s+", " ", content)
|
|
return content
|
|
|
|
|
|
def load_classifier():
|
|
if not os.path.isfile(settings.MODEL_FILE):
|
|
logger.debug(
|
|
"Document classification model does not exist (yet), not "
|
|
"performing automatic matching.",
|
|
)
|
|
return None
|
|
|
|
classifier = DocumentClassifier()
|
|
try:
|
|
classifier.load()
|
|
|
|
except (ClassifierModelCorruptError, IncompatibleClassifierVersionError):
|
|
# there's something wrong with the model file.
|
|
logger.exception(
|
|
"Unrecoverable error while loading document "
|
|
"classification model, deleting model file.",
|
|
)
|
|
os.unlink(settings.MODEL_FILE)
|
|
classifier = None
|
|
except OSError:
|
|
logger.exception("IO error while loading document classification model")
|
|
classifier = None
|
|
except Exception:
|
|
logger.exception("Unknown error while loading document classification model")
|
|
classifier = None
|
|
|
|
return classifier
|
|
|
|
|
|
class DocumentClassifier:
|
|
|
|
# v8 - Added storage path classifier
|
|
FORMAT_VERSION = 8
|
|
|
|
def __init__(self):
|
|
# hash of the training data. used to prevent re-training when the
|
|
# training data has not changed.
|
|
self.data_hash = None
|
|
|
|
self.data_vectorizer = None
|
|
self.tags_binarizer = None
|
|
self.tags_classifier = None
|
|
self.correspondent_classifier = None
|
|
self.document_type_classifier = None
|
|
self.storage_path_classifier = None
|
|
|
|
def load(self):
|
|
with open(settings.MODEL_FILE, "rb") as f:
|
|
schema_version = pickle.load(f)
|
|
|
|
if schema_version != self.FORMAT_VERSION:
|
|
raise IncompatibleClassifierVersionError(
|
|
"Cannot load classifier, incompatible versions.",
|
|
)
|
|
else:
|
|
try:
|
|
self.data_hash = pickle.load(f)
|
|
self.data_vectorizer = pickle.load(f)
|
|
self.tags_binarizer = pickle.load(f)
|
|
|
|
self.tags_classifier = pickle.load(f)
|
|
self.correspondent_classifier = pickle.load(f)
|
|
self.document_type_classifier = pickle.load(f)
|
|
self.storage_path_classifier = pickle.load(f)
|
|
except Exception:
|
|
raise ClassifierModelCorruptError()
|
|
|
|
def save(self):
|
|
target_file = settings.MODEL_FILE
|
|
target_file_temp = settings.MODEL_FILE + ".part"
|
|
|
|
with open(target_file_temp, "wb") as f:
|
|
pickle.dump(self.FORMAT_VERSION, f)
|
|
pickle.dump(self.data_hash, f)
|
|
pickle.dump(self.data_vectorizer, f)
|
|
|
|
pickle.dump(self.tags_binarizer, f)
|
|
|
|
pickle.dump(self.tags_classifier, f)
|
|
pickle.dump(self.correspondent_classifier, f)
|
|
pickle.dump(self.document_type_classifier, f)
|
|
pickle.dump(self.storage_path_classifier, f)
|
|
|
|
if os.path.isfile(target_file):
|
|
os.unlink(target_file)
|
|
shutil.move(target_file_temp, target_file)
|
|
|
|
def train(self):
|
|
|
|
data = list()
|
|
labels_tags = list()
|
|
labels_correspondent = list()
|
|
labels_document_type = list()
|
|
labels_storage_path = list()
|
|
|
|
# Step 1: Extract and preprocess training data from the database.
|
|
logger.debug("Gathering data from database...")
|
|
m = hashlib.sha1()
|
|
for doc in Document.objects.order_by("pk").exclude(
|
|
tags__is_inbox_tag=True,
|
|
):
|
|
preprocessed_content = preprocess_content(doc.content)
|
|
m.update(preprocessed_content.encode("utf-8"))
|
|
data.append(preprocessed_content)
|
|
|
|
y = -1
|
|
dt = doc.document_type
|
|
if dt and dt.matching_algorithm == MatchingModel.MATCH_AUTO:
|
|
y = dt.pk
|
|
m.update(y.to_bytes(4, "little", signed=True))
|
|
labels_document_type.append(y)
|
|
|
|
y = -1
|
|
cor = doc.correspondent
|
|
if cor and cor.matching_algorithm == MatchingModel.MATCH_AUTO:
|
|
y = cor.pk
|
|
m.update(y.to_bytes(4, "little", signed=True))
|
|
labels_correspondent.append(y)
|
|
|
|
tags = sorted(
|
|
tag.pk
|
|
for tag in doc.tags.filter(
|
|
matching_algorithm=MatchingModel.MATCH_AUTO,
|
|
)
|
|
)
|
|
for tag in tags:
|
|
m.update(tag.to_bytes(4, "little", signed=True))
|
|
labels_tags.append(tags)
|
|
|
|
y = -1
|
|
sd = doc.storage_path
|
|
if sd and sd.matching_algorithm == MatchingModel.MATCH_AUTO:
|
|
y = sd.pk
|
|
m.update(y.to_bytes(4, "little", signed=True))
|
|
labels_storage_path.append(y)
|
|
|
|
if not data:
|
|
raise ValueError("No training data available.")
|
|
|
|
new_data_hash = m.digest()
|
|
|
|
if self.data_hash and new_data_hash == self.data_hash:
|
|
return False
|
|
|
|
labels_tags_unique = {tag for tags in labels_tags for tag in tags}
|
|
|
|
num_tags = len(labels_tags_unique)
|
|
|
|
# substract 1 since -1 (null) is also part of the classes.
|
|
|
|
# union with {-1} accounts for cases where all documents have
|
|
# correspondents and types assigned, so -1 isnt part of labels_x, which
|
|
# it usually is.
|
|
num_correspondents = len(set(labels_correspondent) | {-1}) - 1
|
|
num_document_types = len(set(labels_document_type) | {-1}) - 1
|
|
num_storage_paths = len(set(labels_storage_path) | {-1}) - 1
|
|
|
|
logger.debug(
|
|
"{} documents, {} tag(s), {} correspondent(s), "
|
|
"{} document type(s). {} storage path(es)".format(
|
|
len(data),
|
|
num_tags,
|
|
num_correspondents,
|
|
num_document_types,
|
|
num_storage_paths,
|
|
),
|
|
)
|
|
|
|
from sklearn.feature_extraction.text import CountVectorizer
|
|
from sklearn.neural_network import MLPClassifier
|
|
from sklearn.preprocessing import MultiLabelBinarizer, LabelBinarizer
|
|
|
|
# Step 2: vectorize data
|
|
logger.debug("Vectorizing data...")
|
|
self.data_vectorizer = CountVectorizer(
|
|
analyzer="word",
|
|
ngram_range=(1, 2),
|
|
min_df=0.01,
|
|
)
|
|
data_vectorized = self.data_vectorizer.fit_transform(data)
|
|
|
|
# Step 3: train the classifiers
|
|
if num_tags > 0:
|
|
logger.debug("Training tags classifier...")
|
|
|
|
if num_tags == 1:
|
|
# Special case where only one tag has auto:
|
|
# Fallback to binary classification.
|
|
labels_tags = [
|
|
label[0] if len(label) == 1 else -1 for label in labels_tags
|
|
]
|
|
self.tags_binarizer = LabelBinarizer()
|
|
labels_tags_vectorized = self.tags_binarizer.fit_transform(
|
|
labels_tags,
|
|
).ravel()
|
|
else:
|
|
self.tags_binarizer = MultiLabelBinarizer()
|
|
labels_tags_vectorized = self.tags_binarizer.fit_transform(labels_tags)
|
|
|
|
self.tags_classifier = MLPClassifier(tol=0.01)
|
|
self.tags_classifier.fit(data_vectorized, labels_tags_vectorized)
|
|
else:
|
|
self.tags_classifier = None
|
|
logger.debug("There are no tags. Not training tags classifier.")
|
|
|
|
if num_correspondents > 0:
|
|
logger.debug("Training correspondent classifier...")
|
|
self.correspondent_classifier = MLPClassifier(tol=0.01)
|
|
self.correspondent_classifier.fit(data_vectorized, labels_correspondent)
|
|
else:
|
|
self.correspondent_classifier = None
|
|
logger.debug(
|
|
"There are no correspondents. Not training correspondent "
|
|
"classifier.",
|
|
)
|
|
|
|
if num_document_types > 0:
|
|
logger.debug("Training document type classifier...")
|
|
self.document_type_classifier = MLPClassifier(tol=0.01)
|
|
self.document_type_classifier.fit(data_vectorized, labels_document_type)
|
|
else:
|
|
self.document_type_classifier = None
|
|
logger.debug(
|
|
"There are no document types. Not training document type "
|
|
"classifier.",
|
|
)
|
|
|
|
if num_storage_paths > 0:
|
|
logger.debug(
|
|
"Training storage paths classifier...",
|
|
)
|
|
self.storage_path_classifier = MLPClassifier(tol=0.01)
|
|
self.storage_path_classifier.fit(
|
|
data_vectorized,
|
|
labels_storage_path,
|
|
)
|
|
else:
|
|
self.storage_path_classifier = None
|
|
logger.debug(
|
|
"There are no storage paths. Not training storage path classifier.",
|
|
)
|
|
|
|
self.data_hash = new_data_hash
|
|
|
|
return True
|
|
|
|
def predict_correspondent(self, content):
|
|
if self.correspondent_classifier:
|
|
X = self.data_vectorizer.transform([preprocess_content(content)])
|
|
correspondent_id = self.correspondent_classifier.predict(X)
|
|
if correspondent_id != -1:
|
|
return correspondent_id
|
|
else:
|
|
return None
|
|
else:
|
|
return None
|
|
|
|
def predict_document_type(self, content):
|
|
if self.document_type_classifier:
|
|
X = self.data_vectorizer.transform([preprocess_content(content)])
|
|
document_type_id = self.document_type_classifier.predict(X)
|
|
if document_type_id != -1:
|
|
return document_type_id
|
|
else:
|
|
return None
|
|
else:
|
|
return None
|
|
|
|
def predict_tags(self, content):
|
|
from sklearn.utils.multiclass import type_of_target
|
|
|
|
if self.tags_classifier:
|
|
X = self.data_vectorizer.transform([preprocess_content(content)])
|
|
y = self.tags_classifier.predict(X)
|
|
tags_ids = self.tags_binarizer.inverse_transform(y)[0]
|
|
if type_of_target(y).startswith("multilabel"):
|
|
# the usual case when there are multiple tags.
|
|
return list(tags_ids)
|
|
elif type_of_target(y) == "binary" and tags_ids != -1:
|
|
# This is for when we have binary classification with only one
|
|
# tag and the result is to assign this tag.
|
|
return [tags_ids]
|
|
else:
|
|
# Usually binary as well with -1 as the result, but we're
|
|
# going to catch everything else here as well.
|
|
return []
|
|
else:
|
|
return []
|
|
|
|
def predict_storage_path(self, content):
|
|
if self.storage_path_classifier:
|
|
X = self.data_vectorizer.transform([preprocess_content(content)])
|
|
storage_path_id = self.storage_path_classifier.predict(X)
|
|
if storage_path_id != -1:
|
|
return storage_path_id
|
|
else:
|
|
return None
|
|
else:
|
|
return None
|