mirror of
https://github.com/paperless-ngx/paperless-ngx.git
synced 2025-04-09 09:58:20 -05:00
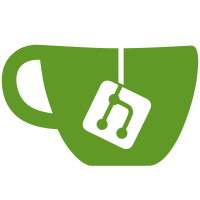
* Saving some start on this * At least partially working for the tesseract parser * Problems with migration testing need to figure out * Work around that error * Fixes max m_pixels * Moving the settings to main paperless application * Starting some consumer options * More fixes and work * Fixes these last tests * Fix max_length on OcrSettings.mode field * Fix all fields on Common & Ocr settings serializers * Umbrellla config view * Revert "Umbrellla config view" This reverts commit fbaf9f4be30f89afeb509099180158a3406416a5. * Updates to use a single configuration object for all settings * Squashed commit of the following: commit 8a0a49dd5766094f60462fbfbe62e9921fbd2373 Author: shamoon <4887959+shamoon@users.noreply.github.com> Date: Tue Dec 19 23:02:47 2023 -0800 Fix formatting commit 66b2d90c507b8afd9507813ff555e46198ea33b9 Author: shamoon <4887959+shamoon@users.noreply.github.com> Date: Tue Dec 19 22:36:35 2023 -0800 Refactor frontend data models commit 5723bd8dd823ee855625e250df39393e26709d48 Author: Adam Bogdał <adam@bogdal.pl> Date: Wed Dec 20 01:17:43 2023 +0100 Fix: speed up admin panel for installs with a large number of documents (#5052) commit 9b08ce176199bf9011a6634bb88f616846150d2b Author: shamoon <4887959+shamoon@users.noreply.github.com> Date: Tue Dec 19 15:18:51 2023 -0800 Update PULL_REQUEST_TEMPLATE.md commit a6248bec2d793b7690feed95fcaf5eb34a75bfb6 Author: shamoon <4887959+shamoon@users.noreply.github.com> Date: Tue Dec 19 15:02:05 2023 -0800 Chore: Update Angular to v17 (#4980) commit b1f6f52486d5ba5c04af99b41315eb6428fd1fa8 Author: shamoon <4887959+shamoon@users.noreply.github.com> Date: Tue Dec 19 13:53:56 2023 -0800 Fix: Dont allow null custom_fields property via API (#5063) commit 638d9970fd468d8c02c91d19bd28f8b0796bdcb1 Author: shamoon <4887959+shamoon@users.noreply.github.com> Date: Tue Dec 19 13:43:50 2023 -0800 Enhancement: symmetric document links (#4907) commit 5e8de4c1da6eb4eb8f738b20962595c7536b30ec Author: shamoon <4887959+shamoon@users.noreply.github.com> Date: Tue Dec 19 12:45:04 2023 -0800 Enhancement: shared icon & shared by me filter (#4859) commit 088bad90306025d3f6b139cbd0ad264a1cbecfe5 Author: Trenton H <797416+stumpylog@users.noreply.github.com> Date: Tue Dec 19 12:04:03 2023 -0800 Bulk updates all the backend libraries (#5061) * Saving some work on frontend config * Very basic but dynamically-generated config form * Saving work on slightly less ugly frontend config * JSON validation for user_args field * Fully dynamic config form * Adds in some additional validators for a nicer error message * Cleaning up the testing and coverage more * Reverts unintentional change * Adds documentation about the settings and the precedence * Couple more commenting and style fixes --------- Co-authored-by: shamoon <4887959+shamoon@users.noreply.github.com>
437 lines
16 KiB
Python
437 lines
16 KiB
Python
import logging
|
|
import os
|
|
import pickle
|
|
import re
|
|
import warnings
|
|
from collections.abc import Iterator
|
|
from datetime import datetime
|
|
from hashlib import sha256
|
|
from pathlib import Path
|
|
from typing import Optional
|
|
|
|
from django.conf import settings
|
|
|
|
from documents.models import Document
|
|
from documents.models import MatchingModel
|
|
|
|
logger = logging.getLogger("paperless.classifier")
|
|
|
|
|
|
class IncompatibleClassifierVersionError(Exception):
|
|
pass
|
|
|
|
|
|
class ClassifierModelCorruptError(Exception):
|
|
pass
|
|
|
|
|
|
def load_classifier() -> Optional["DocumentClassifier"]:
|
|
if not os.path.isfile(settings.MODEL_FILE):
|
|
logger.debug(
|
|
"Document classification model does not exist (yet), not "
|
|
"performing automatic matching.",
|
|
)
|
|
return None
|
|
|
|
classifier = DocumentClassifier()
|
|
try:
|
|
classifier.load()
|
|
|
|
except IncompatibleClassifierVersionError:
|
|
logger.info("Classifier version updated, will re-train")
|
|
os.unlink(settings.MODEL_FILE)
|
|
classifier = None
|
|
except ClassifierModelCorruptError:
|
|
# there's something wrong with the model file.
|
|
logger.exception(
|
|
"Unrecoverable error while loading document "
|
|
"classification model, deleting model file.",
|
|
)
|
|
os.unlink(settings.MODEL_FILE)
|
|
classifier = None
|
|
except OSError:
|
|
logger.exception("IO error while loading document classification model")
|
|
classifier = None
|
|
except Exception: # pragma: no cover
|
|
logger.exception("Unknown error while loading document classification model")
|
|
classifier = None
|
|
|
|
return classifier
|
|
|
|
|
|
class DocumentClassifier:
|
|
# v7 - Updated scikit-learn package version
|
|
# v8 - Added storage path classifier
|
|
# v9 - Changed from hashing to time/ids for re-train check
|
|
FORMAT_VERSION = 9
|
|
|
|
def __init__(self):
|
|
# last time a document changed and therefore training might be required
|
|
self.last_doc_change_time: Optional[datetime] = None
|
|
# Hash of primary keys of AUTO matching values last used in training
|
|
self.last_auto_type_hash: Optional[bytes] = None
|
|
|
|
self.data_vectorizer = None
|
|
self.tags_binarizer = None
|
|
self.tags_classifier = None
|
|
self.correspondent_classifier = None
|
|
self.document_type_classifier = None
|
|
self.storage_path_classifier = None
|
|
|
|
self._stemmer = None
|
|
self._stop_words = None
|
|
|
|
def load(self) -> None:
|
|
# Catch warnings for processing
|
|
with warnings.catch_warnings(record=True) as w:
|
|
with open(settings.MODEL_FILE, "rb") as f:
|
|
schema_version = pickle.load(f)
|
|
|
|
if schema_version != self.FORMAT_VERSION:
|
|
raise IncompatibleClassifierVersionError(
|
|
"Cannot load classifier, incompatible versions.",
|
|
)
|
|
else:
|
|
try:
|
|
self.last_doc_change_time = pickle.load(f)
|
|
self.last_auto_type_hash = pickle.load(f)
|
|
|
|
self.data_vectorizer = pickle.load(f)
|
|
self.tags_binarizer = pickle.load(f)
|
|
|
|
self.tags_classifier = pickle.load(f)
|
|
self.correspondent_classifier = pickle.load(f)
|
|
self.document_type_classifier = pickle.load(f)
|
|
self.storage_path_classifier = pickle.load(f)
|
|
except Exception as err:
|
|
raise ClassifierModelCorruptError from err
|
|
|
|
# Check for the warning about unpickling from differing versions
|
|
# and consider it incompatible
|
|
sk_learn_warning_url = (
|
|
"https://scikit-learn.org/stable/"
|
|
"model_persistence.html"
|
|
"#security-maintainability-limitations"
|
|
)
|
|
for warning in w:
|
|
if issubclass(warning.category, UserWarning):
|
|
w_msg = str(warning.message)
|
|
if sk_learn_warning_url in w_msg:
|
|
raise IncompatibleClassifierVersionError
|
|
|
|
def save(self):
|
|
target_file: Path = settings.MODEL_FILE
|
|
target_file_temp = target_file.with_suffix(".pickle.part")
|
|
|
|
with open(target_file_temp, "wb") as f:
|
|
pickle.dump(self.FORMAT_VERSION, f)
|
|
|
|
pickle.dump(self.last_doc_change_time, f)
|
|
pickle.dump(self.last_auto_type_hash, f)
|
|
|
|
pickle.dump(self.data_vectorizer, f)
|
|
|
|
pickle.dump(self.tags_binarizer, f)
|
|
pickle.dump(self.tags_classifier, f)
|
|
|
|
pickle.dump(self.correspondent_classifier, f)
|
|
pickle.dump(self.document_type_classifier, f)
|
|
pickle.dump(self.storage_path_classifier, f)
|
|
|
|
target_file_temp.rename(target_file)
|
|
|
|
def train(self):
|
|
# Get non-inbox documents
|
|
docs_queryset = Document.objects.exclude(
|
|
tags__is_inbox_tag=True,
|
|
)
|
|
|
|
# No documents exit to train against
|
|
if docs_queryset.count() == 0:
|
|
raise ValueError("No training data available.")
|
|
|
|
labels_tags = []
|
|
labels_correspondent = []
|
|
labels_document_type = []
|
|
labels_storage_path = []
|
|
|
|
# Step 1: Extract and preprocess training data from the database.
|
|
logger.debug("Gathering data from database...")
|
|
hasher = sha256()
|
|
for doc in docs_queryset:
|
|
y = -1
|
|
dt = doc.document_type
|
|
if dt and dt.matching_algorithm == MatchingModel.MATCH_AUTO:
|
|
y = dt.pk
|
|
hasher.update(y.to_bytes(4, "little", signed=True))
|
|
labels_document_type.append(y)
|
|
|
|
y = -1
|
|
cor = doc.correspondent
|
|
if cor and cor.matching_algorithm == MatchingModel.MATCH_AUTO:
|
|
y = cor.pk
|
|
hasher.update(y.to_bytes(4, "little", signed=True))
|
|
labels_correspondent.append(y)
|
|
|
|
tags = sorted(
|
|
tag.pk
|
|
for tag in doc.tags.filter(
|
|
matching_algorithm=MatchingModel.MATCH_AUTO,
|
|
)
|
|
)
|
|
for tag in tags:
|
|
hasher.update(tag.to_bytes(4, "little", signed=True))
|
|
labels_tags.append(tags)
|
|
|
|
y = -1
|
|
sp = doc.storage_path
|
|
if sp and sp.matching_algorithm == MatchingModel.MATCH_AUTO:
|
|
y = sp.pk
|
|
hasher.update(y.to_bytes(4, "little", signed=True))
|
|
labels_storage_path.append(y)
|
|
|
|
labels_tags_unique = {tag for tags in labels_tags for tag in tags}
|
|
|
|
num_tags = len(labels_tags_unique)
|
|
|
|
# Check if retraining is actually required.
|
|
# A document has been updated since the classifier was trained
|
|
# New auto tags, types, correspondent, storage paths exist
|
|
latest_doc_change = docs_queryset.latest("modified").modified
|
|
if (
|
|
self.last_doc_change_time is not None
|
|
and self.last_doc_change_time >= latest_doc_change
|
|
) and self.last_auto_type_hash == hasher.digest():
|
|
return False
|
|
|
|
# substract 1 since -1 (null) is also part of the classes.
|
|
|
|
# union with {-1} accounts for cases where all documents have
|
|
# correspondents and types assigned, so -1 isnt part of labels_x, which
|
|
# it usually is.
|
|
num_correspondents = len(set(labels_correspondent) | {-1}) - 1
|
|
num_document_types = len(set(labels_document_type) | {-1}) - 1
|
|
num_storage_paths = len(set(labels_storage_path) | {-1}) - 1
|
|
|
|
logger.debug(
|
|
"{} documents, {} tag(s), {} correspondent(s), "
|
|
"{} document type(s). {} storage path(es)".format(
|
|
docs_queryset.count(),
|
|
num_tags,
|
|
num_correspondents,
|
|
num_document_types,
|
|
num_storage_paths,
|
|
),
|
|
)
|
|
|
|
from sklearn.feature_extraction.text import CountVectorizer
|
|
from sklearn.neural_network import MLPClassifier
|
|
from sklearn.preprocessing import LabelBinarizer
|
|
from sklearn.preprocessing import MultiLabelBinarizer
|
|
|
|
# Step 2: vectorize data
|
|
logger.debug("Vectorizing data...")
|
|
|
|
def content_generator() -> Iterator[str]:
|
|
"""
|
|
Generates the content for documents, but once at a time
|
|
"""
|
|
for doc in docs_queryset:
|
|
yield self.preprocess_content(doc.content)
|
|
|
|
self.data_vectorizer = CountVectorizer(
|
|
analyzer="word",
|
|
ngram_range=(1, 2),
|
|
min_df=0.01,
|
|
)
|
|
|
|
data_vectorized = self.data_vectorizer.fit_transform(content_generator())
|
|
|
|
# See the notes here:
|
|
# https://scikit-learn.org/stable/modules/generated/sklearn.feature_extraction.text.CountVectorizer.html
|
|
# This attribute isn't needed to function and can be large
|
|
self.data_vectorizer.stop_words_ = None
|
|
|
|
# Step 3: train the classifiers
|
|
if num_tags > 0:
|
|
logger.debug("Training tags classifier...")
|
|
|
|
if num_tags == 1:
|
|
# Special case where only one tag has auto:
|
|
# Fallback to binary classification.
|
|
labels_tags = [
|
|
label[0] if len(label) == 1 else -1 for label in labels_tags
|
|
]
|
|
self.tags_binarizer = LabelBinarizer()
|
|
labels_tags_vectorized = self.tags_binarizer.fit_transform(
|
|
labels_tags,
|
|
).ravel()
|
|
else:
|
|
self.tags_binarizer = MultiLabelBinarizer()
|
|
labels_tags_vectorized = self.tags_binarizer.fit_transform(labels_tags)
|
|
|
|
self.tags_classifier = MLPClassifier(tol=0.01)
|
|
self.tags_classifier.fit(data_vectorized, labels_tags_vectorized)
|
|
else:
|
|
self.tags_classifier = None
|
|
logger.debug("There are no tags. Not training tags classifier.")
|
|
|
|
if num_correspondents > 0:
|
|
logger.debug("Training correspondent classifier...")
|
|
self.correspondent_classifier = MLPClassifier(tol=0.01)
|
|
self.correspondent_classifier.fit(data_vectorized, labels_correspondent)
|
|
else:
|
|
self.correspondent_classifier = None
|
|
logger.debug(
|
|
"There are no correspondents. Not training correspondent "
|
|
"classifier.",
|
|
)
|
|
|
|
if num_document_types > 0:
|
|
logger.debug("Training document type classifier...")
|
|
self.document_type_classifier = MLPClassifier(tol=0.01)
|
|
self.document_type_classifier.fit(data_vectorized, labels_document_type)
|
|
else:
|
|
self.document_type_classifier = None
|
|
logger.debug(
|
|
"There are no document types. Not training document type "
|
|
"classifier.",
|
|
)
|
|
|
|
if num_storage_paths > 0:
|
|
logger.debug(
|
|
"Training storage paths classifier...",
|
|
)
|
|
self.storage_path_classifier = MLPClassifier(tol=0.01)
|
|
self.storage_path_classifier.fit(
|
|
data_vectorized,
|
|
labels_storage_path,
|
|
)
|
|
else:
|
|
self.storage_path_classifier = None
|
|
logger.debug(
|
|
"There are no storage paths. Not training storage path classifier.",
|
|
)
|
|
|
|
self.last_doc_change_time = latest_doc_change
|
|
self.last_auto_type_hash = hasher.digest()
|
|
|
|
return True
|
|
|
|
def preprocess_content(self, content: str) -> str: # pragma: no cover
|
|
"""
|
|
Process to contents of a document, distilling it down into
|
|
words which are meaningful to the content
|
|
"""
|
|
|
|
# Lower case the document
|
|
content = content.lower().strip()
|
|
# Reduce spaces
|
|
content = re.sub(r"\s+", " ", content)
|
|
# Get only the letters
|
|
content = re.sub(r"[^\w\s]", " ", content)
|
|
|
|
# If the NLTK language is supported, do further processing
|
|
if settings.NLTK_LANGUAGE is not None and settings.NLTK_ENABLED:
|
|
import nltk
|
|
from nltk.corpus import stopwords
|
|
from nltk.stem import SnowballStemmer
|
|
from nltk.tokenize import word_tokenize
|
|
|
|
# Not really hacky, since it isn't private and is documented, but
|
|
# set the search path for NLTK data to the single location it should be in
|
|
nltk.data.path = [settings.NLTK_DIR]
|
|
|
|
try:
|
|
# Preload the corpus early, to force the lazy loader to transform
|
|
stopwords.ensure_loaded()
|
|
|
|
# Do some one time setup
|
|
# Sometimes, somehow, there's multiple threads loading the corpus
|
|
# and it's not thread safe, raising an AttributeError
|
|
if self._stemmer is None:
|
|
self._stemmer = SnowballStemmer(settings.NLTK_LANGUAGE)
|
|
if self._stop_words is None:
|
|
self._stop_words = set(stopwords.words(settings.NLTK_LANGUAGE))
|
|
|
|
# Tokenize
|
|
# This splits the content into tokens, roughly words
|
|
words: list[str] = word_tokenize(
|
|
content,
|
|
language=settings.NLTK_LANGUAGE,
|
|
)
|
|
|
|
meaningful_words = []
|
|
for word in words:
|
|
# Skip stop words
|
|
# These are words like "a", "and", "the" which add little meaning
|
|
if word in self._stop_words:
|
|
continue
|
|
# Stem the words
|
|
# This reduces the words to their stems.
|
|
# "amazement" returns "amaz"
|
|
# "amaze" returns "amaz
|
|
# "amazed" returns "amaz"
|
|
meaningful_words.append(self._stemmer.stem(word))
|
|
|
|
return " ".join(meaningful_words)
|
|
|
|
except AttributeError:
|
|
return content
|
|
|
|
return content
|
|
|
|
def predict_correspondent(self, content: str) -> Optional[int]:
|
|
if self.correspondent_classifier:
|
|
X = self.data_vectorizer.transform([self.preprocess_content(content)])
|
|
correspondent_id = self.correspondent_classifier.predict(X)
|
|
if correspondent_id != -1:
|
|
return correspondent_id
|
|
else:
|
|
return None
|
|
else:
|
|
return None
|
|
|
|
def predict_document_type(self, content: str) -> Optional[int]:
|
|
if self.document_type_classifier:
|
|
X = self.data_vectorizer.transform([self.preprocess_content(content)])
|
|
document_type_id = self.document_type_classifier.predict(X)
|
|
if document_type_id != -1:
|
|
return document_type_id
|
|
else:
|
|
return None
|
|
else:
|
|
return None
|
|
|
|
def predict_tags(self, content: str) -> list[int]:
|
|
from sklearn.utils.multiclass import type_of_target
|
|
|
|
if self.tags_classifier:
|
|
X = self.data_vectorizer.transform([self.preprocess_content(content)])
|
|
y = self.tags_classifier.predict(X)
|
|
tags_ids = self.tags_binarizer.inverse_transform(y)[0]
|
|
if type_of_target(y).startswith("multilabel"):
|
|
# the usual case when there are multiple tags.
|
|
return list(tags_ids)
|
|
elif type_of_target(y) == "binary" and tags_ids != -1:
|
|
# This is for when we have binary classification with only one
|
|
# tag and the result is to assign this tag.
|
|
return [tags_ids]
|
|
else:
|
|
# Usually binary as well with -1 as the result, but we're
|
|
# going to catch everything else here as well.
|
|
return []
|
|
else:
|
|
return []
|
|
|
|
def predict_storage_path(self, content: str) -> Optional[int]:
|
|
if self.storage_path_classifier:
|
|
X = self.data_vectorizer.transform([self.preprocess_content(content)])
|
|
storage_path_id = self.storage_path_classifier.predict(X)
|
|
if storage_path_id != -1:
|
|
return storage_path_id
|
|
else:
|
|
return None
|
|
else:
|
|
return None
|